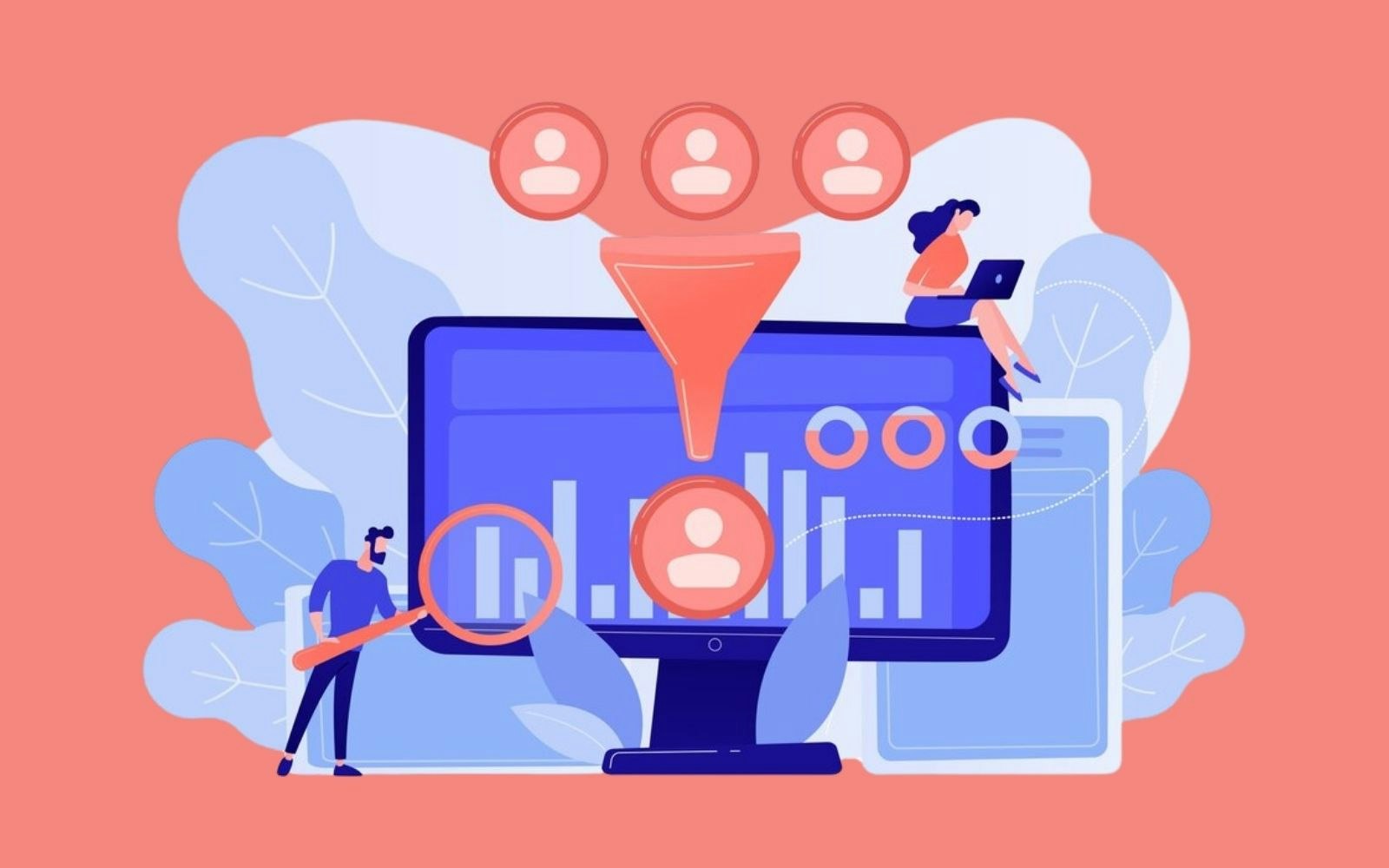
Enriching data to boost performance
7min • Last updated on Apr 3, 2025
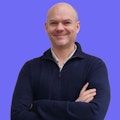
Olivier Renard
Content & SEO Manager
A recent Gartner report reveals an alarming statistic: poor data quality costs businesses an average of $12.9 million per year. Beyond its direct financial impact, incomplete or outdated data distorts analysis, slows decision-making, and complicates marketing strategies.
Yet, companies possess highly valuable information - their customer data. The issue? It is often fragmented, scattered, and underutilised. This is where data enrichment comes in.
Key takeaways:
Data enrichment enhances data quality by filling in missing information and correcting errors.
It benefits all departments, optimising marketing campaigns, improving sales performance, and supporting decision-making.
A methodical approach maximises its impact. Data enrichment relies on key stages and the right tools.
A Customer Data Platform (CDP) unifies and leverages data from multiple sources, simplifying data enrichment with AI-driven insights.
🔎 What is data enrichment (or data appending)? How does it help companies unlock the full potential of their data? Discover its principles, benefits, and best practices for effective implementation. 🚀
What is data enrichment?
Companies collect vast amounts of data, but this information is often incomplete or poorly utilised. As a result, 'dark data' - unexploited information - accounts for 52% of all stored data worldwide.
Data enrichment is the process of improving the quality, usefulness, and depth of raw data by integrating additional information from internal or external sources.
The goal? To obtain more accurate, complete, and actionable insights.
Incomplete or outdated data limits companies’ ability to understand their customers and optimise their strategies. Enrichment enables businesses to:
Personalise marketing campaigns with better audience segmentation.
Improve sales performance by refining lead qualification.
Strengthen decision-making with more complete and reliable data.
Difference with data cleansing
Although complementary, these two processes serve different purposes:
Data cleansing removes or corrects errors in a dataset (e.g., duplicates, incorrect values, outdated information).
Data enrichment adds value to existing data by integrating new relevant information.

Data enrichment vs Data Cleansing
💡 For example, a company can enrich its customer database by adding demographic data (age, gender) or firmographic data (company size, industry) to refine targeting.
DinMo’s identity resolution feature is a good example of data cleansing. It automatically merges customer profiles from multiple sources to create a unified and reliable view — the 'Golden Record'.
Types of data enrichment
Type of enrichment | Description | Example of data |
---|---|---|
Demographic | Personal information used for segmentation and targeting. | Age, gender, family status, income level. |
Firmographic | Business-related data to refine B2B lead qualification. | Industry sector, company size, revenue. |
Behavioural | User interactions and habits to personalise experiences. | Pages visited, purchase frequency, email interactions. |
Geographic | Location-based data on customers or businesses. | Address, time zone, GPS coordinates, IP |
Technographic | Technology used by a company to tailor offers and messaging. | CRM used, marketing tools, cloud infrastructure. |
The different types of enrichment data
👉 Each type of enrichment meets a specific need. Demographic data helps better understand customer profiles, while behavioural data allows companies to optimise marketing campaigns.
💡 An e-commerce platform that enriches its customer profiles with behavioural data (purchase history, pages viewed) can then personalise product recommendations to increase conversion rates.
Operation and tools
Data enrichment follows a multi-step process to improve data quality and relevance by incorporating information from various sources.
Steps and key benefits
1️⃣ Collecting and assessing existing data
What data does the company already have? Where does it come from (CRM, website, apps, customer transactions)? Is it complete and up to date? This analysis helps to identify gaps and define precise data enrichment requirements.
2️⃣ Identifying internal and external data sources
We now need to choose the right sources of enrichment. Some data can be supplemented with internal sources (company databases, transaction histories, insights from a CDP). Others require external sources such as partner datasets, third-party APIs or specialist services.
3️⃣ Cleaning and validating data
Before integrating new information, errors, duplicates, and inconsistencies must be eliminated. Data cleansing ensures the data is usable and relevant. Validation algorithms assist in this process.
4️⃣ Integration and continuous updating
Once enriched, data is stored in a data warehouse, ensuring a single source of truth.
Enrichment is not a one-off task. Since businesses evolve and markets change, data deteriorates over time. Automated updates keep data relevant through real-time synchronisation.
5️⃣ Activation and utilisation
Validated and enriched data becomes a powerful asset for the company. The benefits include:
More precise targeting: Create refined audience segments for personalised marketing campaigns.
Improved decision-making: More reliable analysis for strategic planning.
Enhanced customer relationships: More detailed knowledge of customers, more relevant interactions and better lead prioritisation.
Valuable time savings: Automated processes reduce manual tasks and accelerate insights.
Greater compliance: Robust data governance ensures regulatory compliance (GDPR, CCPA).
👇
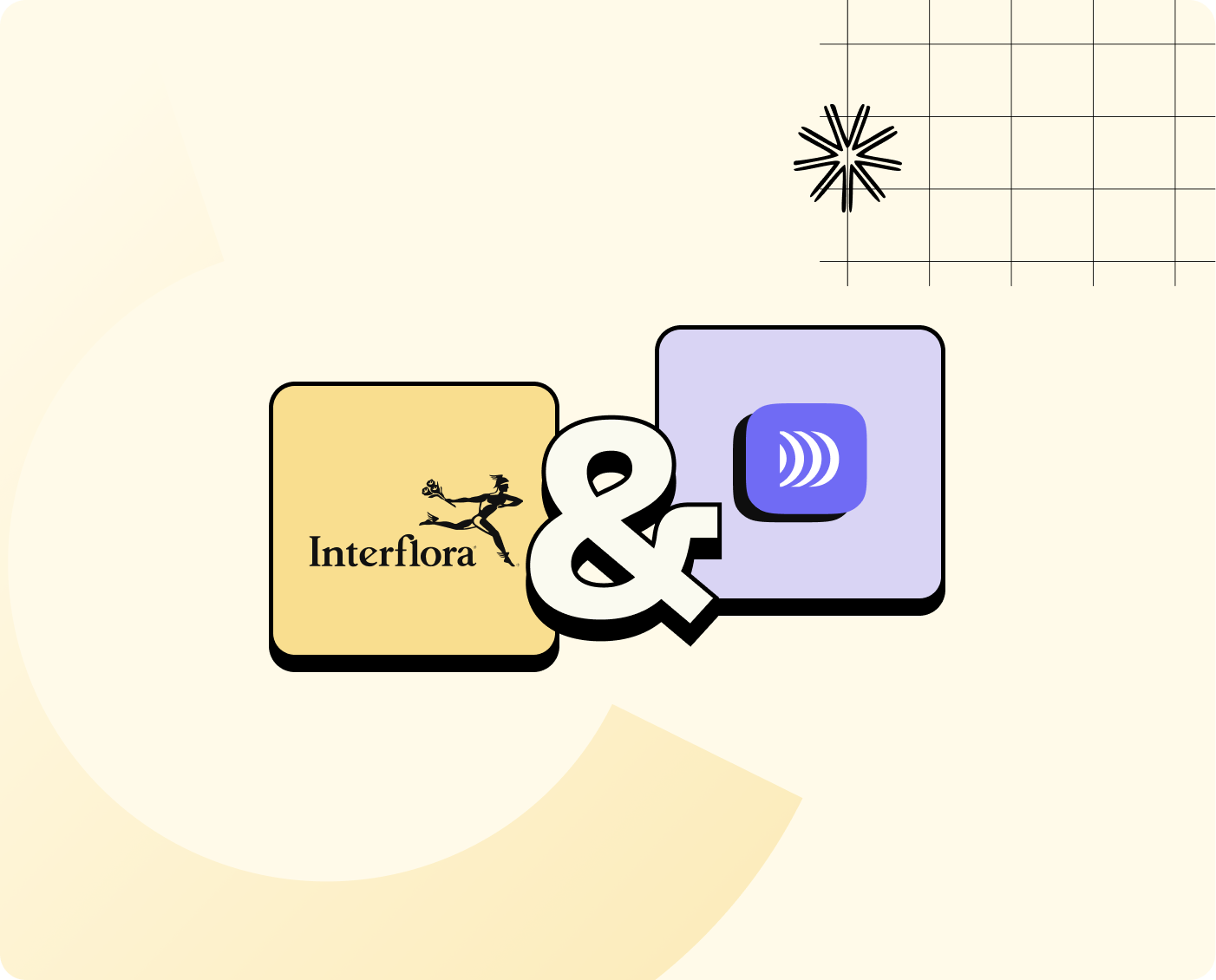
Using data to drive performance: Interflora case study
Data enrichment transforms raw information into a powerful driver of performance across the organisation. A Customer Data Platform is essential for activation. It provides teams with centralised, real-time, actionable data.
Solutions
Different categories of tools enable data enrichment. Here’s an overview of their roles and key features:
Criteria | APIs & Third-Party Databases | Reverse ETL & CDP | CRM & Automation |
---|---|---|---|
Primary Role | Provide external complementary data. | Collect, organise, unify, and enrich data for activation in business tools. | Optimise customer/prospect interactions at every stage of their relationship with the company. |
Type of data | Firmographic, demographic, geographic, technographic. | Behavioural, historical customer data, structured warehouse data, predictive attributes. | Operational data: contact details, sales opportunities, quotes, interactions. |
Use case | Obtain precise company data on prospects (size, industry, revenue). | Create a 360° customer view and distribute enriched data to marketing and sales tools. | Trigger personalised sales alerts or automated email campaigns. |
Main Providers | Clearbit (Breeze), ZoomInfo, Experian, Versium, Apollo. | DinMo, Hightouch, Lytics, Treasure Data. | Salesforce, HubSpot, Marketo, Zoho. |
Solutions for enriching your data
CDP + Reverse ETL solutions centralise, enrich and distribute data to business systems in real time. Let's take a closer look at how they work.
How a CDP enhances data enrichment?
A Customer Data Platform (CDP) is a powerful solution for centralising, enriching, and activating customer data in real time. Unlike a traditional CRM, it gathers data from multiple sources (website, apps, transactions, social media) into a unified customer view.
It encourages better collaboration between the marketing, sales and customer support teams, by reducing internal silos. As a result, each department has a complete and coherent view of the customer.
Our composable CDP goes even further, using machine learning to enrich customer profiles with predictive insights: Future purchase behaviour, intent scoring, advanced segmentation etc.
DinMo’s enrichment features
Predictive attributes: Data is automatically enriched with AI-based predictions, such as customer lifetime value (LTV), churn risk, and product recommendations.
Business rule integration: Our Knowledge Store allows you to add context specific to your business, refining analysis and improving segmentation accuracy.
Custom attribute creation: Custom enrichment with SQL-based or no-code attributes makes it easier to analyse customer behaviour.
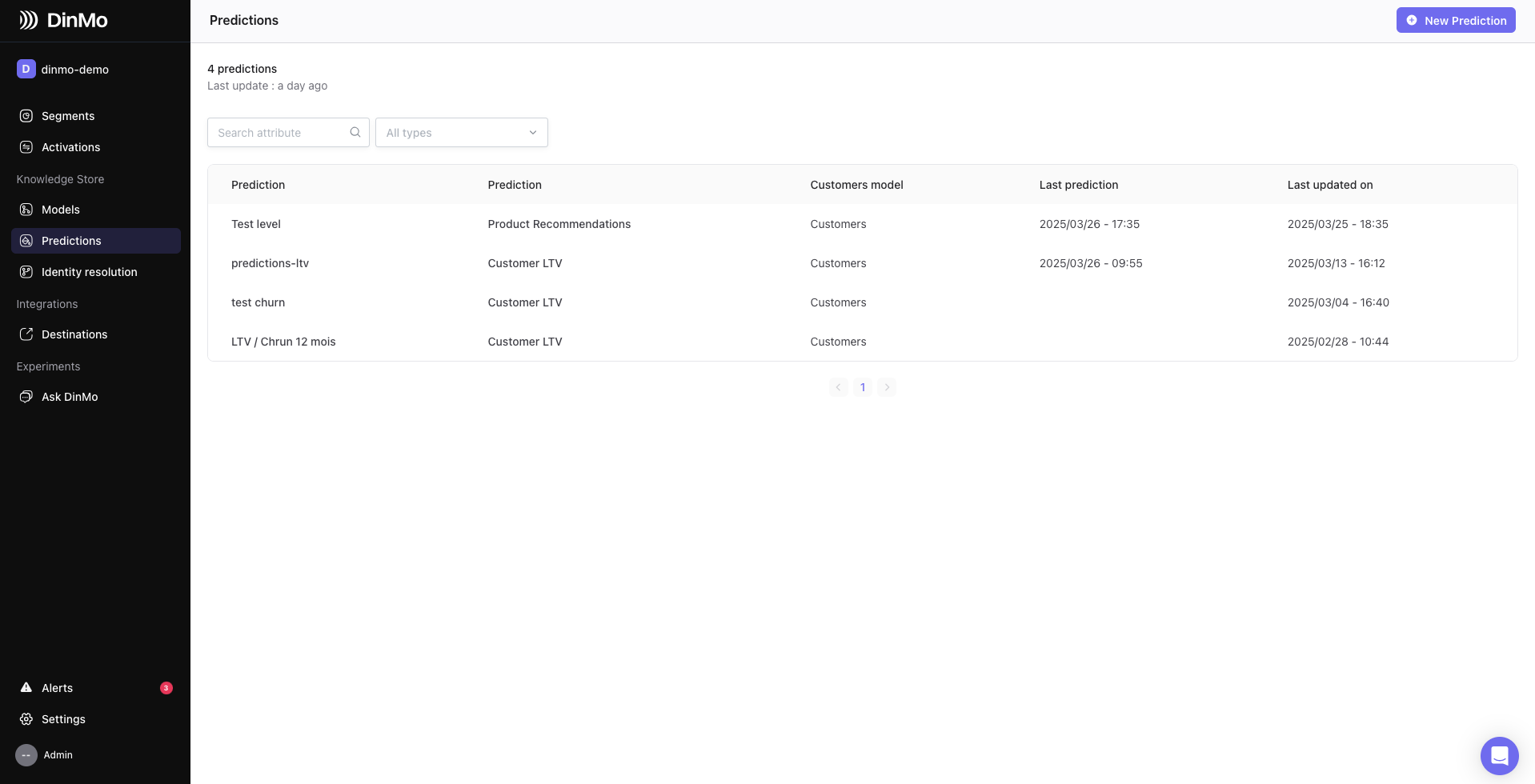
DinMo data enrichment
Successful data enrichment: challenges and best practices
Data enrichment helps improve campaign targeting through finer segmentation and enhanced personalisation. It also reduces customer acquisition costs (CAC) and improves customer loyalty thanks to reliable and accurate data.
Finally, it supports faster, more informed decision-making by enabling higher-quality analysis.
Challenges to anticipate
Always verify the reliability of external sources.
Monitor the cost of enrichment solutions.
Ensure compliance with data protection regulations (GDPR, CCPA).
Automate, while maintaining control over business rules.
Regularly adapt your strategy to meet evolving needs.
Conclusion
Data enrichment is a powerful lever for unlocking the full potential of your customer data. By enhancing your datasets, you deepen customer understanding, optimise campaigns, and accelerate decision-making.
To fully harness the value of this approach, a composable Customer Data Platform is your best ally.
DinMo helps you centralise, enrich, and activate your data in a simple and efficient way, while strengthening collaboration between your marketing, sales, and data teams.
👉 Discover how we can help you make the most of your data!
FAQ
How does data enrichment impact compliance and data governance efforts?
How does data enrichment impact compliance and data governance efforts?
Data enrichment can support compliance and governance when implemented with the right safeguards. It helps organisations keep data accurate, complete, and up to date — key principles under regulations like GDPR. By enriching customer profiles with verified and relevant attributes, businesses reduce the risk of using outdated or incomplete data.
It also improves traceability by creating unified records across systems. However, using third-party sources requires extra care to ensure legal compliance, especially regarding consent and data processing. A clear data governance framework is essential to manage enrichment rules, data origins, and access rights.
What role does Artificial Intelligence play in data enrichment?
What role does Artificial Intelligence play in data enrichment?
Artificial Intelligence (AI) adds a new layer of intelligence to data enrichment. It can analyse large volumes of data and identify patterns or predictions that manual processes would miss.
For example, AI can predict customer lifetime value or churn risk, helping teams prioritise actions. Machine learning algorithms can also recommend relevant content, products, or next steps based on customer behaviour.
These predictive attributes enrich customer profiles and improve decision-making. AI also helps automate enrichment workflows and continuously improve data quality over time.
How can data enrichment improve customer targeting and marketing campaigns?
How can data enrichment improve customer targeting and marketing campaigns?
Data enrichment makes targeting more precise by adding useful attributes to each customer profile. This allows marketers to create detailed audience segments based on behaviour, interests, demographics or company details.
Campaigns can then be tailored to match individual needs, increasing engagement and conversion rates. Enriched data also reduces waste in ad spend, as messages reach more qualified leads. It supports personalisation across channels, from email to paid ads, and helps brands deliver the right message, at the right time, to the right person.